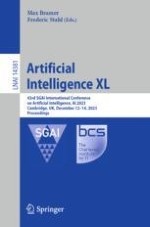
2023 | OriginalPaper | Buchkapitel
On Explanations for Hybrid Artificial Intelligence
verfasst von : Lars Nolle, Frederic Stahl, Tarek El-Mihoub
Erschienen in: Artificial Intelligence XL
Verlag: Springer Nature Switzerland
Aktivieren Sie unsere intelligente Suche, um passende Fachinhalte oder Patente zu finden.
Wählen Sie Textabschnitte aus um mit Künstlicher Intelligenz passenden Patente zu finden. powered by
Markieren Sie Textabschnitte, um KI-gestützt weitere passende Inhalte zu finden. powered by