- 1ICAR- Central Research Institute for Dryland Agriculture (CRIDA), Hyderabad, India
- 2Krishi Vigyan Kendra, Kalaburagi, Karnataka, India
The variability in climate affects the agricultural production especially in drylands. It is necessary to understand and quantify the impacts of resilient technologies as well as effects of extreme events. Keeping these in view, the primary data on household characteristics and the farm income was collected from a sample of 60 farmers each from National Innovations in Climate Resilient Agriculture (NICRA) program adopted village and a comparable control village in the district. The sample was also post classified into small, medium and large farmer to bring out the economic impact across land classes. The impact was estimated following the difference-in-differences (DiD) model as the data allows us to use effectively the data across time and regions. The results show us that the average income of a farm household in the NICRA village is more than 40 percent than non-adopted village and during a drought situation the farmers under NICRA intervention where better off by 19.5 percent. The income from crops and livestock production in adopted village was significantly higher than the control village. The DiD model output showed the farm income of adopted village was 40 percent higher showing that better climate smart interventions improved the farm incomes. The estimate showed that the treated farm household had higher income of Rs. 54,717 than the control during a drought year. Better knowledge and quantification of impact of technology adoption on farm income specially during drought will help to effectively design technological and policy interventions for better drought management in drylands.
Introduction
The agriculture sector is key in reducing food insecurity and is specifically necessary in achieving the major Sustainable Development Goal (SDG 2) “To End hunger achieve global food security, increase people’s nutrition and encourage sustainable agriculture.” It is important to increase the agricultural production and diversity which leads to better farm incomes, which is inevitable to achieve the goal of better nutrition, zero hunger and food inequality (Nkomoki et al., 2018; HLPE, 2020). Agriculture is vulnerable to changing climate and it impacts crop productivity and thereby food security. Climate-related natural disasters are predicted to increase in frequency and magnitude in future and when the agricultural system cannot manage the risks the disasters become hazards and bring substantial loss to the system (IPCC, 2012; Sylvester, 2020). Post disaster assessments in developing countries have shown that 25 percent of economic losses due to climate hazards have direct effect on agriculture. And developing Asian countries are most affected by changing climate and the loss due to rise in temperatures (1.5°C) are estimated to be US$18bn. As Asian countries like India are more reliant on agriculture the damage would be severe with more than 50% directly dependent on agriculture and with about 43 percent of cultivated lands rainfed (Mendelsohn, 2014; Thornton et al., 2014; Food and Agriculture Organization, 2015). Under Indian conditions, the consequences of climate change will be adverse on agriculture and the livelihoods of the farming community with increase on extreme weather events like floods and droughts.
Variability in climate affects agricultural production but effect differs across different region. The climate variability has affected agriculture and has brought about evident changes in crop cultivation patterns in hilly regions of the country and the rainfed regions are also severely affected as they are heavily dependent on rainfall patterns. The major climatic parameters are rainfall and temperature and we have seen that rainfall has tremendous impact on the yield of major staple crops in India (Panda et al., 2019). The semi-arid lands of India represent most of the rainfed and deficit rainfall regions of the country. These make the regions more vulnerable to climate change and deficient rainfall leads to severe drought impacts. The semi-arid regions of the country experience more frequent droughts. The frequency and intensity of drought have been increasing in past years and the changing climate conditions may escalate them. The monsoons in India is important for about 70 percent of the yearly precipitation is received from south west monsoon (June to September months) and a good monsoon brings prosperity to agriculture. Among the major climatic shocks experienced, droughts were found most observed across all regions and locations (Palanisami et al., 2015). Droughts on an average have affected about 79 districts in the country which include 140 million people annually (Bahinipati and Gupta, 2022). Droughts makes vulnerability of the farmers increase and causes other socio-economic problem in rural communities (Lioubimtseva and Henebry, 2009; Bobojonov and Aw-Hassan, 2014; Singh et al., 2014; Chandrasekara et al., 2021; Dhakal et al., 2022).
Indian agriculture is dominated by small and marginal farmers (80%) with the average size of landholding has reduced to 1.16 ha. Nearly 91 percent of households report in India that agriculture is their major source of income with a share of more then 50 percent. Study also showed that, there was increase in share of agriculture to total farm income with increase in landholding (Birthal et al., 2014). Climate change affects especially the smallholders living in the resource constrained arid and semi-arid regions and practising the crop-livestock systems. The contribution and importance of smallholder farming to food production are given special consideration (IFAD and UNEP, 2013; Dey, 2018). Studies show that in South Asia also about 70–80% of farms are smaller than 2 ha and cultivate about 30–40% of the land. And they are more susceptible to changing climate because of their limited land, technological progress, dependence on rain-fed agriculture and increasing poverty (Lowder et al., 2016; Mulwa et al., 2017; Verma and Sudan, 2021). This vulnerability in agricultural production caused by climate change can lead to food insecurity and reduce farmers’ real income. Lack of appropriate quantification of impacts of climate extremes on their incomes would impede the activities to increase their food security (Cervantes-Godoy et al., 2013; Harvey et al., 2018).
Technology and policy interventions are key strategies for influencing how the farmers under scarce land and labour can benefit along with the uncertainties of changing climate. The drylands specially need an inclusive sustainable climate smart agriculture technology and policy. The climate extremes have already put the sustainability of the measures taken to threat, as the effectiveness of development programs are compromised when a region is hit by droughts or floods. Investment in climate smart agriculture and technologies which increase resilience of the sector is imperative for dryland farmers’ adaptation to climate change. It helps farmers reduce the adverse effects of extreme events which have far reaching implications on farm income and food security. Climate resilient crops and management practices help farmers cope with droughts, by minimising the yield loss or by increasing crop yields leading to better incomes to farmers (World Bank, 2008; Gupta et al., 2011; Khatri-Chhetri et al., 2016; Fuglie et al., 2020; Samuel et al., 2022). Adapting to climate hazards and building climate-resilient infrastructure and investing in adaptation measures can avoid farmers from quitting farming. Severe impacts bring uncertainty to agriculture production and thereby farm incomes get drastically reduced (Warner and Afifi, 2014; ILO, 2018).
The National Innovations on Climate Resilient Agriculture (NICRA) is programme of Indian Council of Agricultural Research (ICAR) and it aims at building resilience to Indian agriculture to climate change. And it comprises of components such as strategic research, technology demonstration, sponsored and competitive research grants and capacity building. The Government of India (GOI) has several programmes launched to mitigate and adapt to the impact of climate change. The National Action Plan for Climate Change (NAPCC) was launched in 2008 with 8 major missions and the National Mission for Sustainable Agriculture (NMSA) and the projects under it works in synergy with the NICRA program of the ICAR. This project is one of India’s most comprehensive program and over the years it has great potential in achieving climate resilience especially in times of extreme events like droughts. And it addresses the understanding of the impact of climate change on the drought and floods and evolving suitable adaptation and mitigation strategies (NMSA, 2014). The established programs have been implemented to transform Indian agriculture into a resilient system and have helped to build resilience to climate shocks. This study looks into the impacts of the interventions of the project on the livelihoods of the farm household in the climate resilient villages (CRV) adopted under the same. The CRV was initiated under the Technology Demonstration Component (TDC) of the project in the 151 climatically vulnerable districts of the country. Farmers are adopting the adaptation strategies which have perceived impact on the risk reduction and income enhancement and thereby contributing to food security and poverty reduction. While farmers are adopting, the intensity of adoption, choice of the measures taken and benefits obtained vary with the farm households’ climatic, social, economic and institutional factors. Studies reveal variation in their responses and incomes are influenced by age, education, investment available, credit, resources (land, livestock etc.), institutions and knowledge (Deressa et al., 2009; Below et al., 2012; Ngigi et al., 2017; Diallo et al., 2020). However, micro evidence on the impacts of climate change adaptation techniques on farm income and associated variances is scant. In this research, we try to answer the questions like what is the impact of climate smart technologies and related interventions on income of drylands farm household and quantify the impact of climate hazards like drought and to identify farmer categories more affected to climatic risks. The small holders during a drought not only lose all their meager investments but also end up not able to meet their food needs. Understanding the income effects of adoption of Climate resilient Technologies (CRTs) assume importance towards a more effective drought management at the farm household and higher geographical scales. Keeping these in view, the study attempts to quantify the impact of drought in Kalaburagi district in Karnataka state, its effect on the farm income, and analyses the effect of climate resilient technology adoption on farm household income.
Materials and methods
Study area and research design
The study includes structured household survey, key informant interview and discussions. Since we particularly would like to explore the benefits of adoption of climate smart technologies management strategies as well as the impact of droughts we interviewed the farmers from adopting them versus non-adoption farm households. The study district was purposively selected based on the vulnerability to drought and it is one of the most vulnerable semi-arid districts in India. The treated village is also purposively selected as it is adopted village under the NICRA programme and the control village with similar conditions were selected where no major intervention are implemented. We selected the Kalaburagi district of Karnataka state which is a district with severe water scarcity and frequent droughts (Singh et al., 2018). The sample size included 60 farmers each from the treated and control village randomly selected. The total sample size was 120 farmers. The survey instrument was a well structured pre-tested questionnaire. The farmers were appraised about the purpose of our visit and details of the questionnaire. Comprehensive information on the socio-economic profile of the farm households, land holding particulars, cropping pattern, crop yields and returns, income composition, drought impact on farm income and constraints faced by farmer in improving their income.
Methods
The data were analysed using descriptive statistics, difference in difference (DiD) model and inferential statistics. The descriptive statistics was used to know the socio-economic status of farm household as well as the effects of the variables on the farm income in the treated and control village. The DiD model was used to quantify the impact of climate smart technologies on the farm incomes. Most of the impact studies uses the before-after impact analysis or estimated the outcome or impact of an intervention in the absence of the intervention (Gertler et al., 2016; Abadie and Cattaneo, 2018). The DiD method requires a control group in order to measure the changes in the farm incomes of farmers in the treated village as well as the incomes of farmers in the absence of any intervention and the income changes over time. The unobserved differences in the villages would not be a problem and the method also brings out the changes over time. Therefore, the use of panel based DiD method is one of the important methods of impact evaluation.
The method of difference-in-difference
Regression analysis
Results and discussion
This section gives the description of the sample farmers wherein the socio-economic status, composition of income and employment, details of various crops grown, cropping pattern, cropping intensity, income across different land sizes, impact of climate resilient technologies on the farm income and factors determining the farm income in drylands are being detailed.
Socio-economic characteristics of the sample farmers in the study area
The farmers in the treated and control villages did not differ much in their personal and farm household characteristics. The farmers of the study area belong to average age of 45 years and majority of them belonged to other backward caste. On average the literacy of the farmers was 52 percent, with most of them having pucca Houses. The sample households had an average of 4–5 members with most of them involved in agriculture as their main occupation. About 50 percent of the farmers were involved in livestock rearing, and were able to invest on average Rs. 28,000/acre/year in agriculture. The farm household were well connected to market with good transportation facilities.
Details of the crop cultivation and land holdings in the study area
The agriculture in India, is monsoon dependent and receives monsoon rains from June to September and the major growing seasons are kharif which is during the monsoon period and rabi. The farmers were able to grow at least two crops during the kharif in both the villages. In the treated, we have seen the farmers were able to go for crops in the rabi season also. The major crop in both the villages was rice, followed by cotton and redgram. In rabi crops chilli, mulberry, maize, and vegetables were grown by treated villages farmers. The climate resilient interventions in the treated village enabled them to go for more crops during the rabi. The cropping intensity of the control village was 127.07 percent while the treated village had the cropping intensity of 134 percent. Farmer were growing high value crops like mulberry and vegetables which increase their cropping intensity, leading to better incomes (Table 1).
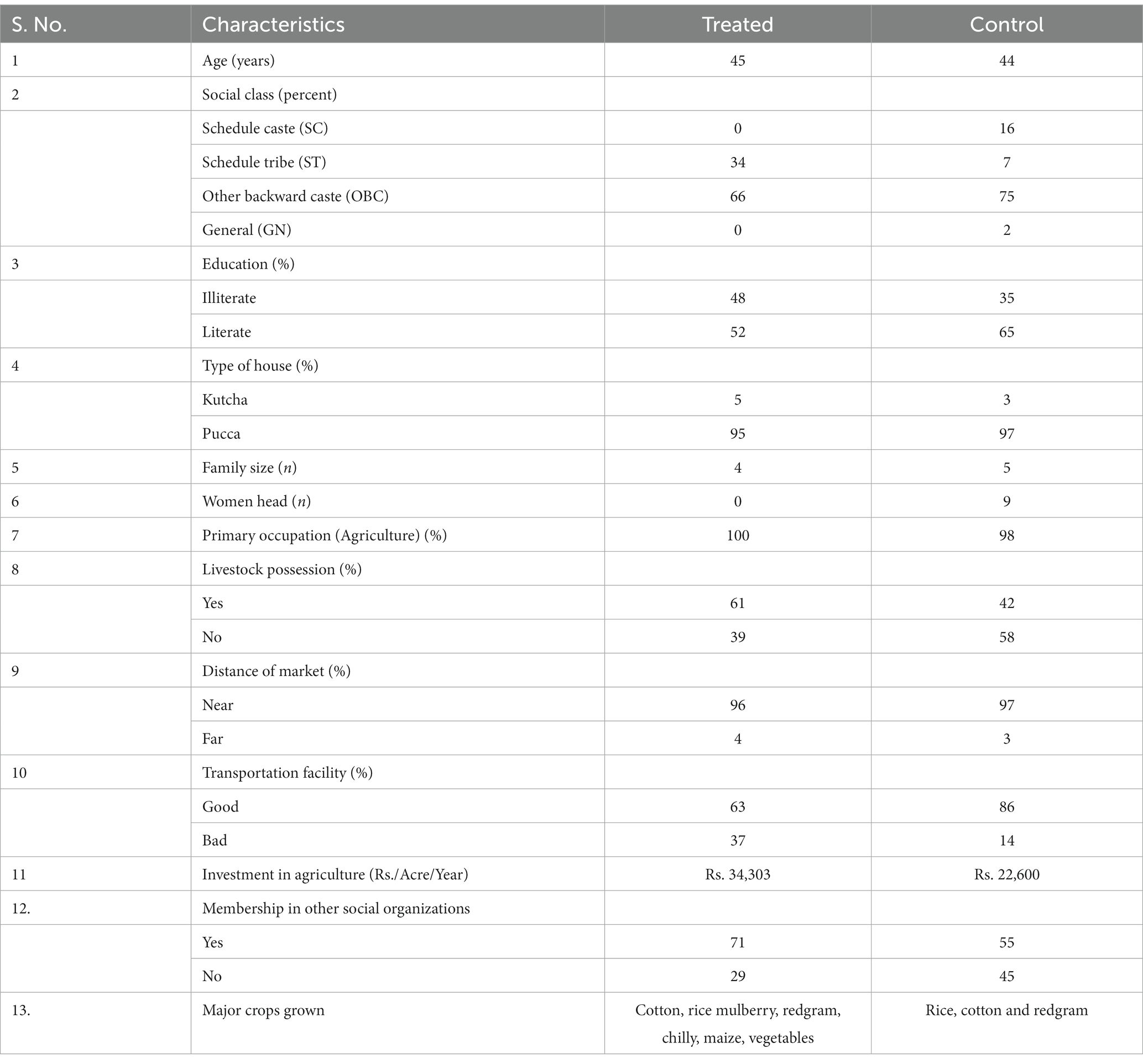
Table 1. Household level descriptive statistics of farmer respondents by treated and control villages.
The farm size available with the farmer is a very important factor for better crop production in agriculture and in India the average landholdings is 1.42 ha (Adamopoulos and Restuccia, 2014; Birthal et al., 2021). In the study area, the average land holding of a farmer was 3.86 ha while the treated village had higher farm size compared to the control village as they had an average of 1.87 ha of leased-in land. Forty percent of the sample farmers were small and marginal farmers, medium and large farmers were about 26 and 35 percent of the total sample. The average size of land holdings in the country is declining and the small and marginal holders account for about 80 percent of the country’s operational holdings and they are more vulnerable to climate risks (Table 2).
Income and employment status of farmers
A farm household’s income comprises of both agricultural and non-agricultural incomes, and crop and livestock were important components. An average dryland farm family’s income composition include income from crops (60–70%), livestock (10–12%) and the rest with non-farm and off- farm income. Income from agriculture includes earnings from cultivation of cereals, pulses, oilseeds, fibres, sugarcane, fruits, vegetables, etc., livestock income includes the farmer earnings from cattle, sheep or goat he is rearing, while off-farm includes income a farmer earns from agricultural wages through labour. And non-farm includes income from small enterprises, kirana shops, tailoring, repairing, and other services (Birthal et al., 2014).
The income composition of farm households in the study area revealed that more than 50 percent of their income came from crop cultivation and 2–8 percent income from livestock rearing. In the treated village, agricultural activities earned the farmer about 82 percent of their total income. It included 74 percent of share coming from crops and 8 percent share of livestock activities. As the farmers in the treated village went for high value crops and vegetable cultivation, the income from crops had the major share. There did not arise a need to diversify their income to other non and off farm sources of income. While in the control village agriculture contributed to only about 55 percent of the income. The off-farm and non-farm income contributed on an average about 43 percent to total FHH income with major share from Non-farm income sources like tailoring, small shops and few were involved in plumbing and electrical works. The treated village could earn a higher share of their income from agriculture through adopting climate smart practices, receiving timely and proper agro-advisories and risk management options (see Figure 1).
Does farm sizes affect the income composition of farm households
The composition of income across different land sizes
Evidences from different studies have differing findings about the relationship of farm sizes and income and the relationship varies with the income sources as well (Adams, 2001; De Janvry et al., 2005; Birthal et al., 2014; Zhu et al., 2022). We tried here to bring out the contribution of different sources of income across farm sizes in the treated and control village. Agriculture is the dominant sources of income in all categories of farm sizes except for the small farmers in the control village where their contribution is below 50 percent (Figure 2). We notice that as farm sizes increases the contribution of agriculture (crop + livestock) to total household income increases especially in the treated village. Livestock rearing is also related to farm size whereas the income from other sources like labour and business activities has an inverse relationship with farm sizes. The small and marginal farmer in the treated village were benefitted, by the climate smart interventions they adopted and their dependence on non-farm businesses is negligible in their total income compared to the control village. The small farmers tend go for low paid labour and small businesses due to their less income from small holdings (see Table 3).
The farmers in the treated village had better employment opportunities in agriculture compared to the control village (Figure 3). On an average a farmer in treated village spent 100 man days more than the control village as the farmers were able to grow 2–3 crops in a year as well as vegetables in the summer. While the employment from non and off-farm were more in the control village. Diversification to different income generating activities and going for off-farm income helps farmers increase their income particularly during times of climatic shocks like droughts (De Janvry et al., 2005; Mishra, 2007; Birthal et al., 2014, 2021).
Impact of climate resilient interventions on farm households
Adoption of climate smart agricultural practices enable the farmers to increase their productivity, diversify their activities and thereby build resilience at times of devastating climatic shocks (Lipper et al., 2014). Interventions through climate outreach programs like NICRA also help in bridging the knowledge and training gaps. Efficient natural resources management is the major contributor towards resilience in the vulnerable villages and efficient conservation of rainfall and moisture are needed for successful crop production. In-situ moisture conservation, supplemental irrigation, water harvesting and efficiently utilising the harvested water, green manuring etc., are given priority in NICRA villages and these technologies are being demonstrated in the villages also. In the study village, under the project farm ponds were constructed in farmer field, one community farm pond, one check dam also was constructed, and the desilting of the drainage channel was also taken up which resulted in increase of water storage potential of the village. It helped farmers to provide supplemental irrigation to pigeon pea, mulberry, banana and green gram and supplemental irrigation to pigeon pea during dry spell fetched an additional yield of 2.5 q/ha. The harvested water from farm ponds helped farmers increase cropping intensity and thereby improved their incomes (Reddy et al., 2018). Climate smart practices bring about various benefits to farmers (Hansen et al., 2018; Ogada et al., 2020), among which the better indicator is the farm incomes which is the sum of incomes obtained from agricultural and non-agricultural activities.
The role of climate smart interventions on agricultural productivity and income of farm households adopting them compared to farmers not benefiting is analysed through descriptive statistics, difference in difference methodology and multiple linear regression model.
Impact on crop productivity and returns
The treated villages were growing a number of crops both in the kharif and rabi seasons. The average productivity of main crops has increased significantly compared to the control village. There was notable increase in productivity of crops with adoption and the highest increase was for maize crop (50%), followed by banana (8%), sunflower (7.14%), cotton (4.6%), redgram (4.34%) and jowar (1.33%). The net returns from different crops cultivated was higher in the treated village, and farmers on an average earned about Rs. 15,000 per crop (Table 4).
Impact of the interventions on farm income and employment in the treated village
The adoption of climate smart practices have become inevitable under the changing climatic scenario as it improves the agricultural productivity, increases the income of farmers and meets the food and nutritional security of the growing population (Rama Rao et al., 2017; Ojo and Baiyegunhi, 2020; Dhakal et al., 2022). Farm household incomes were higher incomes after the interventions from the project.
The difference in the average farm incomes before and after the project interventions was Rs. 84,770/FHH/year. An agriculture household’s income significantly varies with their land holding and therefore we also tried to quantify the benefits of climate interventions across farm sizes. The small farmers were benefitted the most from the intervention followed by the medium and large farmers. Small farmers had about 80 percentage increase, while the income of medium and larger farmers increased by about 40 percent (see Table 5).
Impact on income composition and farm sizes
The composition wise change in the farm incomes across farm sizes were also studied. The impact of intervention across different farm sizes showed that large farmers received Rs. 1,20,000/FHH more income when compared to their income before the intervention and the small farmers were benefitted more than the medium farmers with an average significant increase in their farm incomes (Figure 4).
To increase farm incomes and to achieve food security it is necessary to target climate smart practices in almost every village (Issahaku and Abdulai, 2019; Ojo and Baiyegunhi, 2020; Malhi et al., 2021; Ali et al., 2022). The benefits of climate smart interventions and adoption of the same by the farmers was quantified using the difference in difference model (DiD). The income gains were captured using DiD where the difference in the Income before and after interventions was taken as the first difference and second difference as the income change between the control and treated village. The results are depicted in the Figure 5. A farm household which adopts climate smart practises earns on an average nearly Rs. 2,50,000/year compared to the control village. The first difference in the farm incomes across the villages before intervention was Rs. 45,705 after intervention it was Rs. 74,767/farm household/year. The difference in differences was found to Rs. 29,062 and it captures the important differences in the incomes and removes biases which can arise because of trends and inherent differences in the study locations.
Drought impact on the farm household income and benefits of adoption of climate resilient technologies
India stands third after China and US for being affected by highest number of natural disasters over the past 20 years, and among them drought is an important climatic shock but there are few studies on the various quantifiable post impact assessment (Kala, 2017; Bahinipati, 2020; Mohanty, 2020).
The total farm household income during a drought year was compared to a normal year across interventions. The treated village farmers income from agriculture was higher than the control village. During a drought year, farmers face a major decline in their incomes especially from agricultural activities. Figure 6 depicts the income obtained from agriculture during normal and drought year. An average farmer in the treated village had more than 90 percent higher income from agriculture both in normal as well as in drought year. And the reduction in the income from agriculture during drought is more in the control village than the treated village farmers. Droughts have devastating impact on farm families but in the treated village farmers are better off due to the climate resilient interventions and practices.
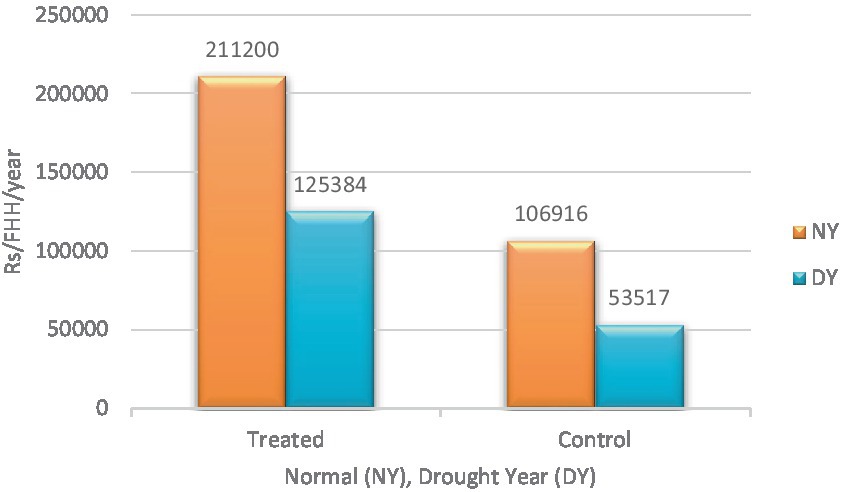
Figure 6. Drought impact on income from agriculture and benefits of adoption of climate resilient technologies.
During climate extremes farm households try to diversify their incomes, it can be seen from the figure that notable differences are there in the composition of FHH income between drought year and normal year. The doughnut chart represents the changes in composition of income during drought in Figure 7. The outer doughnut is the income during a drought year and inner a normal year. The income from crops and livestock activities reduced by 12 percent due to drought while contribution of non-farm activities to total incomes increased by 10 percent. Droughts significantly affect the crop yields and there reduce the farm incomes, farmers go for diversifying their incomes by taking up non- farm activities to sustain (Singh et al., 2018).
Climate change adaptation brings substantial increase in profits to farmers and reduction in risk associated to climate extremes. The loss a farm household avoided during a drought year by climate smart agriculture practices was quantified through the DID approach. The double difference estimated was Rs. 54,717/FHH/year, a farmer in the treated village is able to minimise his loss during drought by better adaptation strategies. Climate smart interventions in the form of technologies, trainings, agro-advisories etc., increases the profitability of farming through higher yields, reduction in loss and thereby enhanced incomes. And the adoption at the farm level is imperative to mitigate the loss in productivity and farm incomes (Mishra, 2007; Nambi et al., 2015).
Factors influencing the farm income in drylands
The income of a farm household is dependent on different socio-economic, climatic, institutional and technological factors under the changing climate the success and effectiveness of an adaptation strategy to be adopted by a farm household depends on the various factors. And it is imperative to know and quantify the impact of the various factors. From the result of the regression analysis, we see that the major significant factors affecting FHH were farm size, livestock possession, whether they adopt climate smart practices, their social status, and investment in agriculture (Mamun et al., 2021). The land a farmer possess is the most important asset and results reveal that an acre increase in his land will increase his income by Rs. 23,353 and a farmer who possess livestock can increase his income by Rs. 43,836. Livestock always plays an important part in the income composition of small and resource poor farmers, the sale of animals and their products are major income generating activities. And also farm families with livestock are more resilient to climate change (Aryal et al., 2018; Ogada et al., 2020; Kuchimanchi et al., 2021). The results also indicate that the social class of farmer and family size had impacts on incomes. The socially disadvantaged castes are economically disadvantaged may be due to factor like poor resource endowment, exclusion for publics networks and extension services (Krishna et al., 2019; Ruzzante et al., 2021). Adoption of climate smart technologies significantly increase incomes especially with changing climate and frequency of climatic hazards. The results indicate that a benefit of Rs. 39,867/FHH is earned by a farmer in the treated village compared to the control village farmer. The education of farmer and family size were not significant factors in determining the income of the farmers (see Figure 8).
Conclusion and policy implications
Our estimation of impact of climate change on farm income unlike most studies focuses on composition of farm income, impact across farm sizes and as well as quantifying the impact of intervention especially climate resilient technologies. The results reveal that farm households with interventions have more than 60 percent share of their income from agriculture. And the average operational land holdings was higher compared to the control village as farmers were able to lease in land in the treated villages. The livestock component contributed about 10 percent to the total household income. The analysis of farm incomes across the farm sizes reveal that the contribution of agriculture to total income increases with farm size. In the village with climate resilient interventions the small farmers did not go for non-farm income sources unlike the farmers in the control village. The productivity increases in the crops increased from 5 to 50 percent across different crops through climate resilient interventions compared to the crop yield in the control village. The difference in the average farm incomes before and after the project interventions was Rs. 84,770/FHH/year and most benefitted were the small farmers. And DiD estimated was Rs. 26,062/FHH/year during a normal year.
The impact of climate extremes like drought on farm income across farm sizes and composition were quantified. There was significant reduction in income of farmers during drought especially in the control village. Income from crop activities has the major loss while the share of income from non-farm activities increased. Farmers tend to go for small businesses, non-agriculture labour etc., to meet their needs. The double difference estimated for drought was Rs. 54,717/FHH/year and farmers in the treated village were able to minimise their loss during drought by better adaptation strategies. The study also brings out the impact of the various socio-economic and institutional factors which affect the farm incomes in drylands. The farm size, adoption of climate resilient technologies, livestock possession, family size and investment in agriculture were found to be the significant contributors to farm incomes.
The barriers in technology adoption like uncertainties about its performance and costs involved can be overcome by the formation of climate resilient villages. The technology demonstration component of the program builds confidence in farming community come forward to adopt resilient technologies. These adaptation strategies are devised for risk reduction, especially risk related to climate like droughts, floods and diseases/pests and they minimize the loss and help to sustain the farm incomes. The adoption of adaptation strategies, diversification, water management, along with insurance have the potential to reduce the loss in farm incomes in times of climate extremes (De Janvry et al., 2005; Fuglie et al., 2020). Development of climate smart agriculture which protects the natural resources and ecosystem are needed. Policies are needed for a productive as well as resilient system to maintain food production under changing climates. Mainstreaming the climate adaptation practices/strategies through livelihood programs, rural employment generation schemes would help in building the resilience of farm households against climate extremes.
Data availability statement
The raw data supporting the conclusions of this article will be made available by the authors, without undue reservation.
Ethics statement
Ethical review and approval was not required for the study on human participants in accordance with the local legislation and institutional requirements. Written informed consent from the patients/participants or patients/participants legal guardian/next of kin was not required to participate in this study in accordance with the national legislation and the institutional requirements.
Author contributions
JS: Conceptualization, Formal analysis, Methodology, Writing – original draft. CR: Supervision, Validation, Writing – review & editing. Pushpanjali_ Data curation, Writing – review & editing. AB: Data curation, Writing – review & editing. BR: Data curation, Writing – review & editing. AR: Writing – review & editing. NK: Data curation, Writing – review & editing. AK: Writing – review & editing. VS: Project administration, Resources, Writing – review & editing. MP: Project administration, Resources, Writing – review & editing. SG: Data curation, Writing – review & editing. RT: Resources, Writing – review & editing.
Funding
The author(s) declare that no financial support was received for the research, authorship, and/or publication of this article.
Acknowledgments
The authors acknowledge the support given by NICRA. We are thankful to the staff of Krishi Vigyan Kendra, Kalaburagi and to the farmers in the study villages who shared their information. The authors are also thankful to the reviewers for their constructive comments.
Conflict of interest
The authors declare that the research was conducted in the absence of any commercial or financial relationships that could be construed as a potential conflict of interest.
Publisher’s note
All claims expressed in this article are solely those of the authors and do not necessarily represent those of their affiliated organizations, or those of the publisher, the editors and the reviewers. Any product that may be evaluated in this article, or claim that may be made by its manufacturer, is not guaranteed or endorsed by the publisher.
References
Abadie, A., and Cattaneo, M. D. (2018). Econometric methods for program evaluation. Annu. Rev. Econ. 10, 465–503. doi: 10.1146/annurev-economics-080217-053402
Adamopoulos, T., and Restuccia, D. (2014). The size distribution of farms and international productivity differences. Am. Econ. Rev. 104, 1667–1697. doi: 10.1257/aer.104.6.1667
Adams, R. H. Jr.. (2001). Non-farm income, inequality and poverty in rural Egypt and Jordan. Policy research working paper 2572. World Bank, Washington, DC
Ali, H., Menza, M., Hagos, F., and Haileslassie, A. (2022). Impact of climate-smart agriculture adoption on food security and multidimensional poverty of rural farm households in the Central Rift Valley of Ethiopia. Agric. Food Secur. 11:62. doi: 10.1186/s40066-022-00401-5
Aryal, J. P., Rahut, D. B., Maharjan, S., and Erenstein, O. (2018). Factors affecting the adoption of multiple climate-smart agricultural practices in the Indo-Gangetic Plains of India. Nat. Resour. Forum 42, 141–158. doi: 10.1111/1477-8947.12152
Bahinipati, C. S. (2020). Assessing the costs of droughts in rural India: a comparison of economic and non-economic loss and damage. Curr. Sci. 118, 1832–1841. doi: 10.18520/cs/v118/i11/1832-1841
Bahinipati, C. S., and Gupta, A. K. (2022). Methodological challenges in assessing loss and damage from climate-related extreme events and slow onset disasters: evidence from India. Int. J. Disaster Risk Reduct. 83:103418. doi: 10.1016/j.ijdrr.2022.103418
Below, T. B., Mutabazi, K. D., Kirschke, D., Franke, C., Sieber, S., Siebert, R., et al. (2012). Can farmers’ adaptation to climate change be explained by socio-economic household-level variables? Glob. Environ. Change 22, 223–235. doi: 10.1016/j.gloenvcha.2011.11.012
Birthal, P. S., Hazrana, J., and Negi, D. S. (2021). Effectiveness of farmers’ risk management strategies in smallholder agriculture: evidence from India. Clim. Change 169:30. doi: 10.1007/s10584-021-03271-1
Birthal, P. S., Negi, D. S., Jha, A. K., and Singh, D. (2014). Income sources of farm households in India: determinants, distributional consequences and policy implications. Agric. Econ. Res. Rev. 27, 37–48. doi: 10.5958/j.0974-0279.27.1.003
Bobojonov, I., and Aw-Hassan, A. (2014). Impacts of climate change on farm income security in Central Asia: an integrated modelling approach. Agric. Ecosyst. Environ. 188, 245–255. doi: 10.1016/j.agee.2014.02.033
Cervantes-Godoy, D., Kimura, S., and Antón, J. (2013). “Smallholder risk management in developing countries” in OECD food, agriculture and fisheries papers, No. 61 (Paris: OECD Publishing)
Chandrasekara, S. S., Kwon, H. H., Vithanage, M., Obeysekera, J., and Kim, T. W. (2021). Drought in South Asia: a review of drought assessment and prediction in South Asian countries. Atmosphere 12:369. doi: 10.3390/atmos12030369
De Janvry, A., Sadoulet, E., and Zhu, N. (2005). The role of non-farm incomes in reducing rural poverty and inequality in China working paper 1001. Department of Agricultural & Resource Economics, University of California, Berkley. Available at: http://repositories.cdlib.org/are_ucb
Deressa, T. T., Hassan, R. M., Ringler, C., Alemu, T., and Yesuf, M. (2009). Determinants of farmers’ choice of adaptation methods to climate change in the Nile Basin of Ethiopia. Glob. Environ. Change 19, 248–255. doi: 10.1016/j.gloenvcha.2009.01.002
Dey, S. (2018). The role of employment diversification in reducing vulnerability to poverty among marginal and small-holder agricultural households in India. Margin J. Appl. Econ. Res. 12, 88–112. doi: 10.1177/0973801017740661
Dhakal, C., Khadka, S., Park, C., and Escalante, C. L. (2022). Climate change adaptation and its impacts on farm income and downside risk exposure. Resour. Environ. Sustain. 10:100082. doi: 10.1016/j.resenv.2022.100082
Diallo, A., Donkor, E., and Owusu, V. (2020). Climate change adaptation strategies, productivity and sustainable food security in southern Mali. Clim. Change 159, 309–327. doi: 10.1007/s10584-020-02684-8
Food and Agriculture Organization. Climate change and food security: risks and responses. FAO Rome. (2015). Available at: http://www.fao.org/3/a-i5188e.pdf
Fuglie, K., Gautam, M., Goyal, A., and Maloney, W. F.. (2020). Harvesting prosperity: technology and productivity growth in agriculture. Word Bank, Washington, DC
Gertler, P. J., Martinez, S., Premand, P., Rawlings, L. B., and Vermeersch, C. M. (2016). Impact evaluation in practice Washington, DC: World Bank Publications.
Gupta, A., Tyagi, P., and Sehgal, V. K. (2011). Drought disaster challenges and mitigation in India: strategic appraisal. Curr. Sci. 100, 1795–1806.
Hansen, J., Hellin, J., Rosenstock, T., Fisher, E., Cairns, J., Stirling, C., et al. (2018). Climate risk management and rural poverty reduction. Agric. Syst. 172, 28–46. doi: 10.1016/j.agsy.2018.01.019
Harvey, C. A., Saborio-Rodríguez, M., Martinez-Rodríguez, M. R., Viguera, B., Chain-Guadarrama, A., Vignola, R., et al. (2018). Climate change impacts and adaptation among smallholder farmers in Central America. Agric. Food Secur. 7:57. doi: 10.1186/s40066-018-0209-x
HLPE. (2020). Food security and nutrition: building a global narrative towards 2030. High Level Panel of Experts on Food Security and Nutrition of the Committee on World Food Security. Rome, 191. Available at: http://www.fao.org/3/ca9731en/ca9731en.pdf
IFAD and UNEP. Smallholders, food security and the environment. Rome: International Fund for Agricultural Development, 29. (2013).
ILO. (2018). The employment impact of climate change adaptation. Input document for the G20 Climate Sustainability Working Group. International Labour Office (ILO), Geneva
IPCC (2012) in Managing the risks of extreme events and disasters to advance climate change adaptation: special report of the Intergovernmental Panel on Climate Change. eds. C. B. Field, V. Barros, T. F. Stocker, D. Qin, D. J. Dokken, and K. L. Ebi, et al. (Cambridge, MA: Cambridge University Press), 582.
Issahaku, G., and Abdulai, A. (2019). Adoption of climate-smart practices and its impact on farm performance and risk exposure among smallholder farmers in Ghana. Aust. J. Agric. Resour. Econ. 64, 396–420. doi: 10.1111/1467-8489.12357
Kala, C. P. (2017). Environmental and socioeconomic impacts of drought in India: lessons for drought management. Appl. Ecol. Environ. Sci. 5:43. doi: 10.12691/aees-5-2-3
Khatri-Chhetri, A., Aryal, J. P., Sapkota, T. B., and Khurana, R. (2016). Economic benefits of climate-smart agricultural practices to smallholder farmers in the Indo-Gangetic Plains of India. Curr. Sci. 110, 1251–1256. doi: 10.18520/cs/v110/i7/1251-1256
Krishna, V. V., Aravalath, L. M., and Vikraman, S. (2019). Does caste determine farmer access to quality information? PLoS One 14:e0210721. doi: 10.1371/journal.pone.0210721
Kuchimanchi, B. R., Van Paassen, A., and Oosting, S. J. (2021). Understanding the vulnerability, farming strategies and development pathways of smallholder farming systems in Telangana, India. Clim. Risk Manag. 31:100275. doi: 10.1016/j.crm.2021.100275
Lioubimtseva, E., and Henebry, G. M. (2009). Climate and environmental change in arid Central Asia: impacts, vulnerability, and adaptations. J. Arid Environ. 73, 963–977. doi: 10.1016/j.jaridenv.2009.04.022
Lipper, L., Thornton, P., Campbell, B. M., Baedeker, T., Braimoh, A., Bwalya, M., et al. (2014). Climate-smart agriculture for food security. Nat. Clim. Change 4, 1068–1072. doi: 10.1038/nclimate2437
Lowder, S. K., Skoet, J., and Raney, T. (2016). The number, size, and distribution of farms, smallholder farms, and family farms worldwide. World Dev. 87, 16–29. doi: 10.1016/j.worlddev.2015.10.041
Malhi, G. S., Kaur, M., and Kaushik, P. (2021). Impact of climate change on agriculture and its mitigation strategies: a review. Sustainability 13:1318. doi: 10.3390/su13031318
Mamun, A. A., Roy, S., Islam, A. R. M. T., Alam, G. M. M., Alam, E., Chandra Pal, S., et al. (2021). Smallholder farmers’ perceived climate-related risk, impact, and their choices of sustainable adaptation strategies. Sustainability 13:11922. doi: 10.3390/su132111922
Mendelsohn, R. (2014). The impact of climate change on agriculture in Asia. J. Integr. Agric. 13, 660–665. doi: 10.1016/S2095-3119(13)60701-7
Mishra, S. (2007). Household livelihood and coping mechanism during drought among Oraon tribe of Sundargarh District of Orissa, India. J. Soc. Sci. 15, 181–186. doi: 10.1080/09718923.2007.11892580
Mohanty, A. (2020). Preparing India for extreme climate events, (December), 68 Available at: https://www.ceew.in/publications/preparing-india-extreme-climate-events.
Mulwa, C., Marenya, P., and Kassie, M. (2017). Response to climate risks among smallholder farmers in Malawi: a multivariate probit assessment of the role of information, household demographics, and farm characteristics. Clim. Risk Manag. 16, 208–221. doi: 10.1016/j.crm.2017.01.002
Nambi, A. A., Bahinipati, C. S., Raghunath, R., and Nagendran, R. (2015). Farm household level adaptation metrics for agriculture and water sectors. Int. J. Clim. Change Strateg. Manag. 7, 27–40. doi: 10.1108/IJCCSM-04-2013-0048
Ngigi, M. W., Mueller, U., and Birner, R. (2017). Gender differences in climate change adaptation strategies and participation in group-based approaches: an intra-household analysis from rural Kenya. Ecol. Econ. 138, 99–108. doi: 10.1016/j.ecolecon.2017.03.019
Nkomoki, W., Bavorová, M., and Banout, J. (2018). Adoption of sustainable agricultural practices and food security threats: effects of land tenure in Zambia. Land Use Policy 78, 532–538. doi: 10.1016/j.landusepol.2018.07.021
NMSA (2014). National mission for sustainable agriculture, operational guidelines Department of Agriculture & Cooperation, Ministry of Agriculture Government of India Available at: https://agricoop.nic.in/Documents/Final_guidelines_3.pdf.
Ogada, M. J., Rao, E. J. O., Radeny, M., Recha, J. W., and Solomon, D. (2020). Climate-smart agriculture, household income and asset accumulation among smallholder farmers in the Nyando basin of Kenya. World Dev. Perspect. 18:100203. doi: 10.1016/j.wdp.2020.100203
Ojo, T. O., and Baiyegunhi, L. J. S. (2020). Determinants of climate change adaptation strategies and its impact on the net farm income of rice farmers in South-West Nigeria. Land Use Policy 95:103946. doi: 10.1016/j.landusepol.2019.04.007
Palanisami, K., Haileslassie, A., Kakumanu, K. R., Ranganathan, C. R., Wani, S. P., Craufurd, P., et al. (2015). “Climate change, gender and adaptation strategies in dryland systems of South Asia: a household level analysis in Andhra Pradesh, Karnataka and Rajasthan states of India” in ICRISAT research program resilient dryland system research report No. 65. Patancheru, Telangana, India: ICRISAT
Panda, A., Sahu, N., Behera, S., Sayama, T., Sahu, L., Avtar, R., et al. (2019). Impact of climate variability on crop yield in Kalahandi, Bolangir, and Koraput districts of Odisha, India. Climate 7:126. doi: 10.3390/cli7110126
Rama Rao, C. A., Raju, B. M. K., Subba Rao, A. V. M., Rao, K. V., Samuel, J., Kausalya Ramachandran, K., et al. (2017). Assessing vulnerability and adaptation of agriculture to climate change in Andhra Pradesh. Ind. J. Agri. Econ. 72, 375–384.
Reddy, K. S., Prasad, J. V. N. S., Osman, M., Ramana, D. B. V., Nagasree, K., Rejani, R., et al. (2018). “Technology demonstrations: enhancing resilience and adaptive capacity of farmers to climate variability” in National innovations in climate resilient agriculture (NICRA) project (Hyderabad: ICAR-Central Research Institute for Dryland Agriculture), 129.
Ruzzante, S., Labarta, R., and Bilton, A. (2021). Adoption of agricultural technology in the developing world: a meta-analysis of the empirical literature. World Dev. 146:105599. doi: 10.1016/j.worlddev.2021.105599
Samuel, J., Rao, C. A. R., Raju, B. M. K., Reddy, A. A., Pushpanjali,, AGK, R., et al. (2022). Assessing the impact of climate resilient technologies in minimizing drought impacts on farm incomes in drylands. Sustainability 14:382. doi: 10.3390/su14010382
Singh, N. P., Bantilan, C., and Byjesh, K. (2014). Vulnerability and policy relevance to drought in the semi-arid tropics of Asia – a retrospective analysis. Weather. Clim. Extremes 3, 54–61. doi: 10.1016/j.wace.2014.02.002
Singh, C., Rahman, A., Srinivas, A., and Bazaz, A. (2018). Risks and responses in rural India: implications for local climate change adaptation action, climate. Risk Manage. 21, 52–68. doi: 10.1016/j.crm.2018.06.001
Sylvester, O. (2020). “Achieving food security in the face of inequity, climate change, and conflict” in The difficult task of peace (Cham: Palgrave Macmillan), 277–295.
Thornton, P. K., Ericksen, P. J., Herrero, M., and Challinor, A. J. (2014). Climate variability and vulnerability to climate change: a review. Glob. Change Biol. 20, 3313–3328. doi: 10.1111/gcb.12581
Verma, S., and Sudan, F. K. (2021). Impact of climate change on marginal and small farmers’ livelihood and their adaptation strategies-a review. Reg. Econ. Dev. Res. 2, 96–112. doi: 10.37256/redr.222021896
Warner, K., and Afifi, T. (2014). Where the rain falls: evidence from 8 countries on how vulnerable households use migration to manage the risk of rainfall variability and food insecurity. Clim. Dev. 6, 1–17. doi: 10.1080/17565529.2013.835707
World Bank. Climate change impacts in drought and flood affected areas: case studies in India. World Bank report. (2008), 148. Available at: http://hdl.handle.net/10986/8075. (Accessed December 10, 2021).
Keywords: climate, dryland, agriculture, farm income, resilience, India, drought
Citation: Samuel J, Rama Rao CA, Pushpanjali, Anshida Beevi CN, Raju BMK, Amarender Reddy A, Nagarjuna Kumar R, Reddy AGK, Singh VK, Prabhakar M, Siva GS and Teggelli RG (2024) Enhancing farm income resilience through climate smart agriculture in drought-prone regions of India. Front. Water. 6:1327651. doi: 10.3389/frwa.2024.1327651
Edited by:
André Rodrigues, Federal University of Minas Gerais, BrazilReviewed by:
Alfred Owuor Opere, University of Nairobi, KenyaNetrananda Sahu, University of Delhi, India
Copyright © 2024 Samuel, Rama Rao, Pushpanjali, Anshida Beevi, Raju, Amarender Reddy, Nagarjuna Kumar, Reddy, Singh, Prabhakar, Siva and Teggelli. This is an open-access article distributed under the terms of the Creative Commons Attribution License (CC BY). The use, distribution or reproduction in other forums is permitted, provided the original author(s) and the copyright owner(s) are credited and that the original publication in this journal is cited, in accordance with accepted academic practice. No use, distribution or reproduction is permitted which does not comply with these terms.
*Correspondence: Josily Samuel, josilysamuel@gmail.com