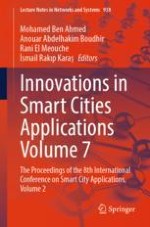
2024 | OriginalPaper | Buchkapitel
Plant Disease Classification and Segmentation Using a Hybrid Computer-Aided Model Using GAN and Transfer Learning
verfasst von : Khaoula Taji, Yassine Taleb Ahmad, Fadoua Ghanimi
Erschienen in: Innovations in Smart Cities Applications Volume 7
Verlag: Springer Nature Switzerland
Aktivieren Sie unsere intelligente Suche, um passende Fachinhalte oder Patente zu finden.
Wählen Sie Textabschnitte aus um mit Künstlicher Intelligenz passenden Patente zu finden. powered by
Markieren Sie Textabschnitte, um KI-gestützt weitere passende Inhalte zu finden. powered by