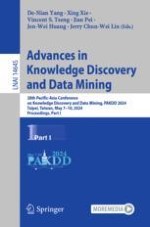
2024 | OriginalPaper | Buchkapitel
Spatial-Temporal Transformer with Error-Restricted Variance Estimation for Time Series Anomaly Detection
verfasst von : Yuye Feng, Wei Zhang, Haiming Sun, Weihao Jiang
Erschienen in: Advances in Knowledge Discovery and Data Mining
Verlag: Springer Nature Singapore
Aktivieren Sie unsere intelligente Suche, um passende Fachinhalte oder Patente zu finden.
Wählen Sie Textabschnitte aus um mit Künstlicher Intelligenz passenden Patente zu finden. powered by
Markieren Sie Textabschnitte, um KI-gestützt weitere passende Inhalte zu finden. powered by